Zoom Seminar: Exploring Automatic Assessment of Spoken-Language Interpreting: Comparing N-Gram-, Neural-, and LLM-based Metrics
Seminar
Date
September 09, 2024 (Mon)
Mode
Meeting ID: 912 4012 6713
Passcode: 855469
Time
12:45 PM - 1:45 PM
Speaker
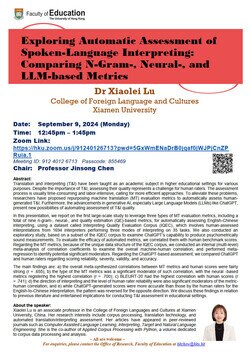
Exploring Automatic Assessment of Spoken-Language Interpreting: Comparing N-Gram-, Neural-, and LLM-based Metrics
Dr Xiaolei Lu
College of Foreign Language and Cultures
Xiamen University
Date: September 9, 2024 (Monday)
Time: 12:45pm – 1:45pm
Zoom Link:
Meeting ID: 912 4012 6713 Passcode: 855469
Chair: Professor Jinsong Chen
Abstract:
Translation and interpreting (T&I) have been taught as an academic subject in higher educational settings for various purposes. Despite the importance of T&I, assessing their quality represents a challenge for human raters. The assessment process is usually time-consuming and labor-intensive, calling for more efficient approaches. To alleviate these problems, researchers have proposed repurposing machine translation (MT) evaluation metrics to automatically assess human-generated T&I. Furthermore, the advancements in generative AI, especially Large Language Models (LLMs) like ChatGPT, present new possibilities of automating assessment of T&I quality.
In this presentation, we report on the first large-scale study to leverage three types of MT evaluation metrics, including a total of nine n-gram-, neural-, and quality estimation (QE)-based metrics, for automatically assessing English-Chinese interpreting, using a dataset called Interpreting Quality Evaluation Corpus (IQEC), which involves human-assessed interpretations from 1694 interpreters performing three modes of interpreting on 35 tasks. We also conducted an exploratory study, based on a subset of the IQEC corpus to examine ChatGPT’s capability to produce psychometrically sound measurements. To evaluate the efficacy of automated metrics, we correlated them with human benchmark scores. Regarding the MT metrics, because of the unique data structure of the IQEC corpus, we conducted an internal (multi-level) meta-analysis of correlation coefficients to examine the overall machine-human correlation, and performed meta-regression to identify potential significant moderators. Regarding the ChatGPT-based assessment, we compared ChatGPT and human raters regarding scoring reliability, severity, validity, and accuracy.
The main findings are: a) the overall meta-synthesized correlations between MT metrics and human scores were fairly strong (r = .655); b) the type of the MT metrics was a significant moderator of such correlation, with the neural -based metrics registering the highest correlation (r = .700); c) BLEURT-20 had the highest correlation with human scores (r = .741); d) the direction of interpreting and the level of human rater reliability were also significant moderators of the metric-human correlation; and e) while ChatGPT-generated scores were more accurate than those by the human raters for the English-to-Chinese interpretation, the pattern was reversed for the opposite direction. We discuss these findings in relation to previous literature and entertained implications for conducting T&I assessment in educational settings.
About the speaker:
Xiaolei Lu is an associate professor in the College of Foreign Languages and Cultures at Xiamen University, China. Her research interests include corpus processing, translation technology, and automated translation/interpreting assessment. Her articles have appeared in peer-reviewed journals such as Computer Assisted Language Learning, Interpreting, Target and Natural Language Engineering. She is the co-author of Applied Corpus Processing with Python, a volume dedicated to corpus data processing and analysis.
~ All are welcome ~
For enquiries, please contact the Office of Research, Faculty of Education at hkchow@hku.hk